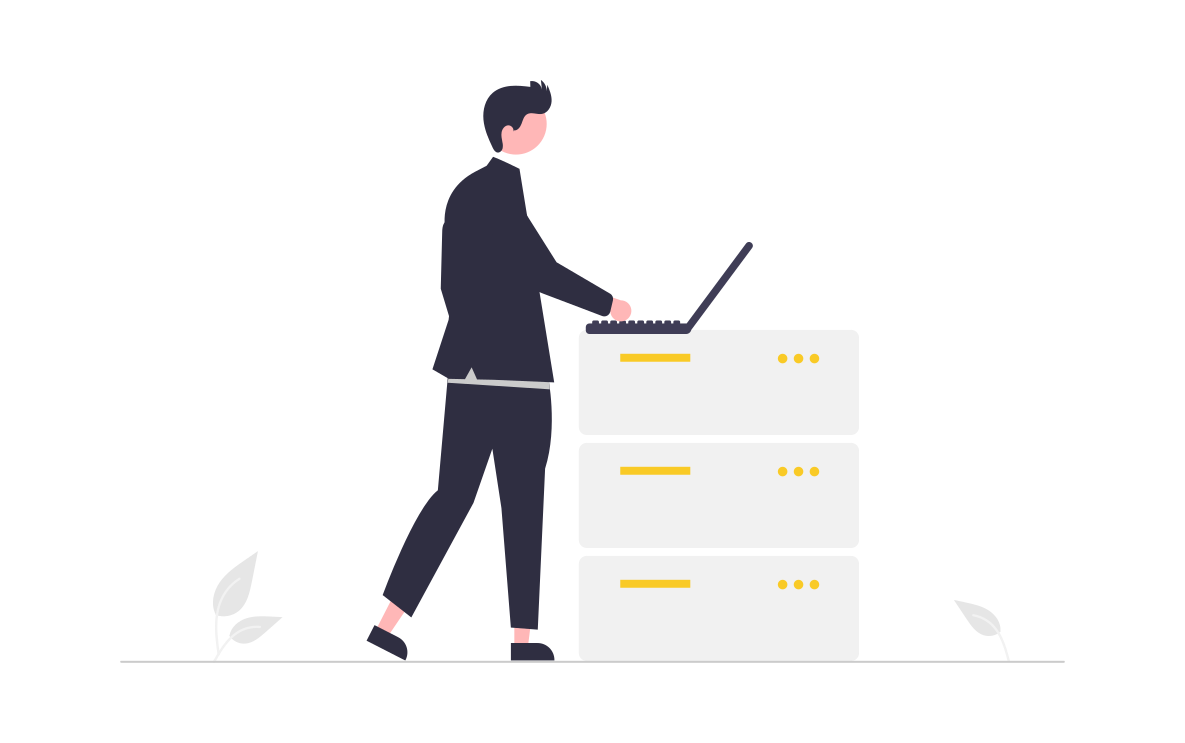
In today's data-driven world, gathering information from the web has become a crucial aspect of various industries, ranging from e-commerce and market research to content aggregation and competitive analysis. Scrapy, a powerful and flexible web scraping framework, has gained popularity for its ability to streamline the process of collecting data from websites. When combined with MongoDB, a versatile NoSQL database, Scrapy becomes an even more potent tool for storing and managing scraped data efficiently. In this blog post, we'll explore the benefits and steps involved in using Scrapy with MongoDB for effective data scraping.
Before installing any dependency, I advise you to create a virtualenv, using:
virtualenv env
Lets start by installing scrapy and pymongo:
pip install scrapy pymongo
Now we create a new scrapy project
scrapy startproject mongoTest
For this example we will scrape books.toscrape.com collecting title, link, image, price and create an item for it by adding item class name BookItem in items.py
import scrapy
class BookItem(scrapy.Item):
title = scrapy.Field()
price = scrapy.Field()
link = scrapy.Field()
image = scrapy.Field()
And for that, let's create an new spider named `books` and edit it using the following commands
scrapy genspider books "https://books.toscrape.com"
scrapy edit books
I will assume that you already know how to use pagination and xpaths for this, so the final code would be something like this:
import scrapy
from ..items import BookItem
class BooksSpider(scrapy.Spider):
name = "books"
allowed_domains = ["https://books.toscrape.com/"]
start_urls = ["https://books.toscrape.com/catalogue/page-%d.html" %
x for x in range(1, 51)]
def parse(self, response):
items = response.xpath("//section/div/ol[@class='row']/li")
for item in items:
book = BookItem()
book['title'] = item.xpath(".//h3/a/@title").get()
book['price'] = item.xpath(
".//p[@class='price_color']/text()").get()
book['link'] = "https://books.toscrape.com/catalogue/" + \
item.xpath(".//h3/a/@href").get()
book['image'] = "https://books.toscrape.com" + \
item.xpath(".//img[@class='thumbnail']/@src").get()[2:]
yield book
after crawl testing we can see that all books are scraped without any errors. Now, we need to create a pipeline to save data into mongodb by adding BookPipeline class into pipelines.py
from itemadapter import ItemAdapter
import pymongo
from scrapy.exceptions import DropItem
MONGO_URI = "mongodb uri retrieved from account settings"
class BookPipeline:
def __init__(self):
# start a new connection to mongodb server
self.conn = pymongo.MongoClient(MONGO_URI)
self.db = self.conn.test # specify the database to work on
def process_item(self, item, spider):
# create an adapter to facilitate data iteration
adapter = ItemAdapter(item)
# collection is basically like a table in sql
collection = self.db['books']
valid = True
for data in item:
if not data: # we check for data validity in the item
valid = False
# raise error by droping item
raise DropItem("Missing {0}!".format(data))
if valid:
# we try to find the item in db
# using link as unique identifier
# and update it.
# If None is returned, we insert it as new
if collection.find_one_and_update(
{"link": adapter['link']},
{"$set": dict(item)}) is None:
collection.insert_one(dict(item))
else:
print("item updated")
return item
def close_spider(self, spider):
# we close the connection once all scraping comes to an end
self.conn.close()
After that, we simply add the pipeline in settings.py
# Configure item pipelines
# See https://docs.scrapy.org/en/latest/topics/item-pipeline.html
ITEM_PIPELINES = {
"mongoTest.pipelines.BookPipeline": 300,
}
Results:
to check data in mongo db, I am using mongodb compass on ubuntu, and here is the scraped data listed in our books collection:
Conclusion:
Mongodb provides a great and new technology for storing scraped data and gives an easy structure to avoid duplicates and update data on the run, you might find some scrape time differences which you can always enhance using async programming.